Learning Analytics for Annotated Courses
When teachers and students use Hypothesis annotation to explore course readings, certain benefits are immediately obvious. When students find one another in the margins of documents they tell us that the experience of reading is less lonely, which matters now more than ever. When teachers and students discuss key passages, their conversation — linked directly to highlights, displayed in the margin — is more focused than in conventional forums.
This experience of social reading can be delivered as an assignment in a learning management system (LMS). Students are expected to read a chapter of a book and make a prescribed number of substantive annotations; teachers grade the exercise.
Or it can happen less formally. A teacher, who may or may not operate in the context of an LMS, can use annotation to open a window into students’ minds, whether or not their annotations influence their grades.
All these scenarios produce data. The annotated corpus has, at its core, a set of documents. Annotation data explicitly records highlighted passages in documents along with the notes linked to those highlights. It also records conversations that flow from those annotated highlights
Teachers and students, as well as authors and publishers of course readings, can all learn useful things from this data. Teachers and students may want to know who has participated and how often, which are the most highlighted passages across all documents in the course, or which highlights have attracted the longest conversation threads. Authors and publishers will also want to know which passages have attracted the most highlights and discussion.
The annotation data can also support deeper analysis. How often do teachers or students ask questions? How often are questions answered, or not? How often do teachers ask questions answered by students, students ask questions answered by teachers, or students ask questions answered by students?
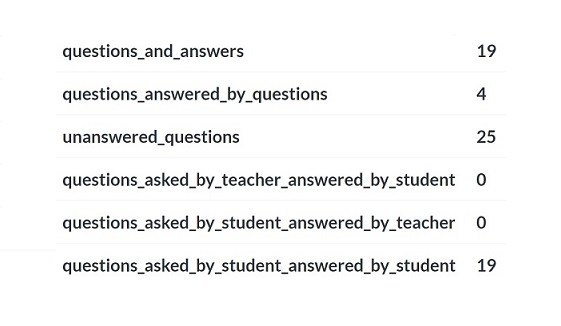
We are providing these views now, on an experimental basis, in order to explore what learning analytics can become in the realm of annotated course reading. Surfacing questions asked by students and answered by students, for example, could be a way to measure the sort of peer interaction that research suggests can improve engagement and outcomes.
Of course the devil is always in the details. Our initial naive approach looks for question marks in annotations, then correlates responses to those annotations. This is convenient because it works with natural discourse, but imprecise because questions are often rhetorical.
A less convenient but more precise approach would require participants to signal intent using an inline hashtag, or a formal tag on the annotation.
A convenient and precise approach would bake affordances into the annotation tool. For example, there might be reaction icons to say things like:
– I’m asking a question that expects an answer
– I’m confused on this point
– I’m highlighting an example of a rhetorical device
In my view we can’t know, a priori, what those baked-in affordances should be. We’ll need to discover them in collaboration with teachers who are using Hypothesis in their courses, and who are willing and able to explore these ideas before they can be fully codified in software.
If you’re a teacher using Hypothesis actively this term, and you’d like to participate in this research, please let us know. We’ll invite you to try our prototype analytics system and help us evolve it.